The AI-revolution in UX design
A new era on ways of working
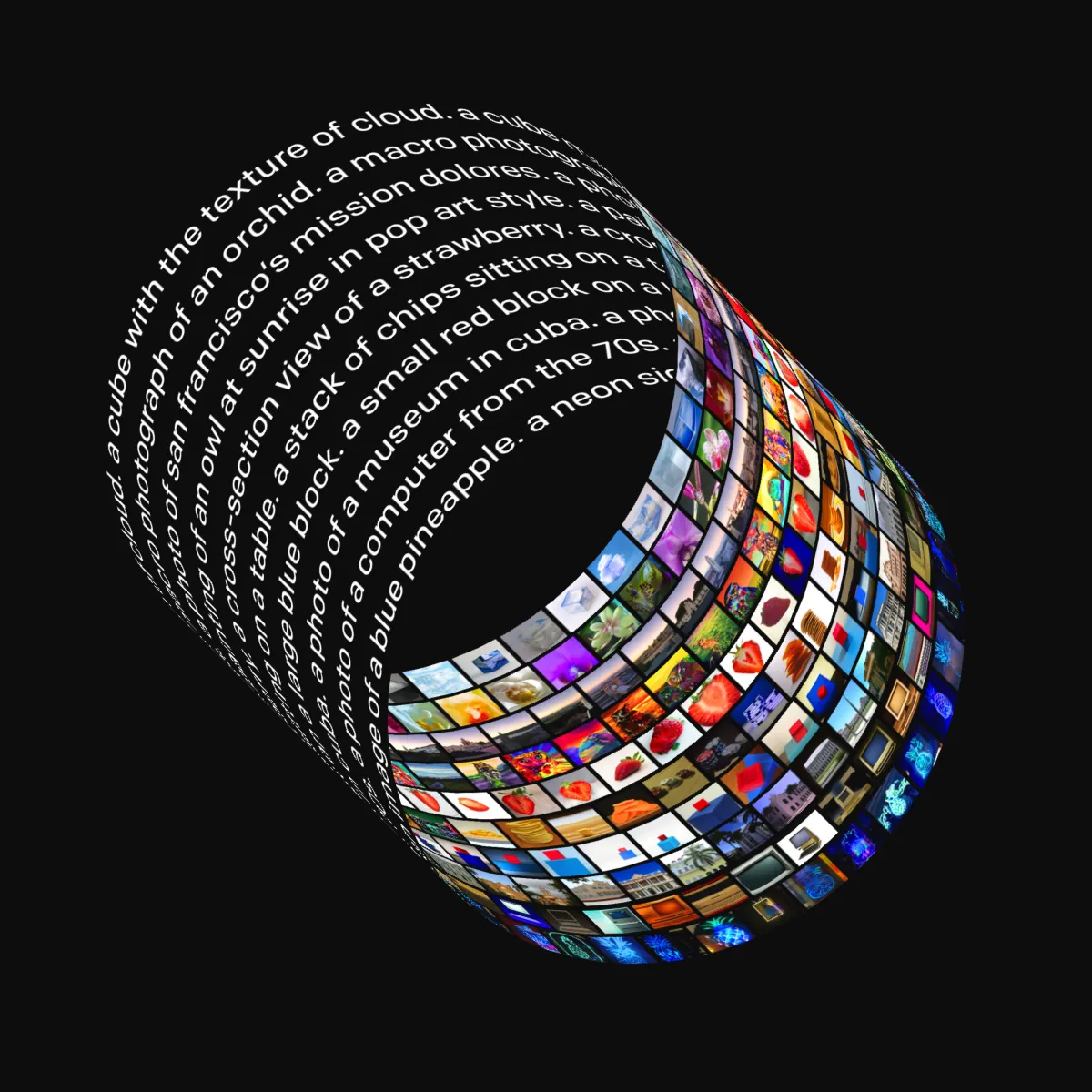
Happy Sunday. "Don't worry, you'll hit 'em over the head with data." This quote was a highlight of my week. Inspiring me to hit y'all over the head with my perspective on AI and UX.
The Evolution
We're experiencing a seismic shift in UX and product development. The growing importance of AI as a feature is evident in Apple's recent announcement, showcasing seamless integration of AI into their core OS. No one has produced a good AI as a product… yet.
The most successful AI features solve real user problems and integrate naturally within existing workflows. As AI becomes more intelligent, it must also become more human-centered, ethical, and aligned with user needs.
I see many designers playing with AI-powered features and tools, but that only scratched the surface. Plugins will continuously evolve, while it's helpful to shave a bit of time from your workflow, it doesn't hit the fundamentals deeply.
Design is the key differentiator.
Let's explore 3 key considerations for AI-driven UX design strategies.
- A New Paradigm: The AI Development Cycle.
- Value Migration in AI: Generalist vs. Specialist Model
- The Role of Design
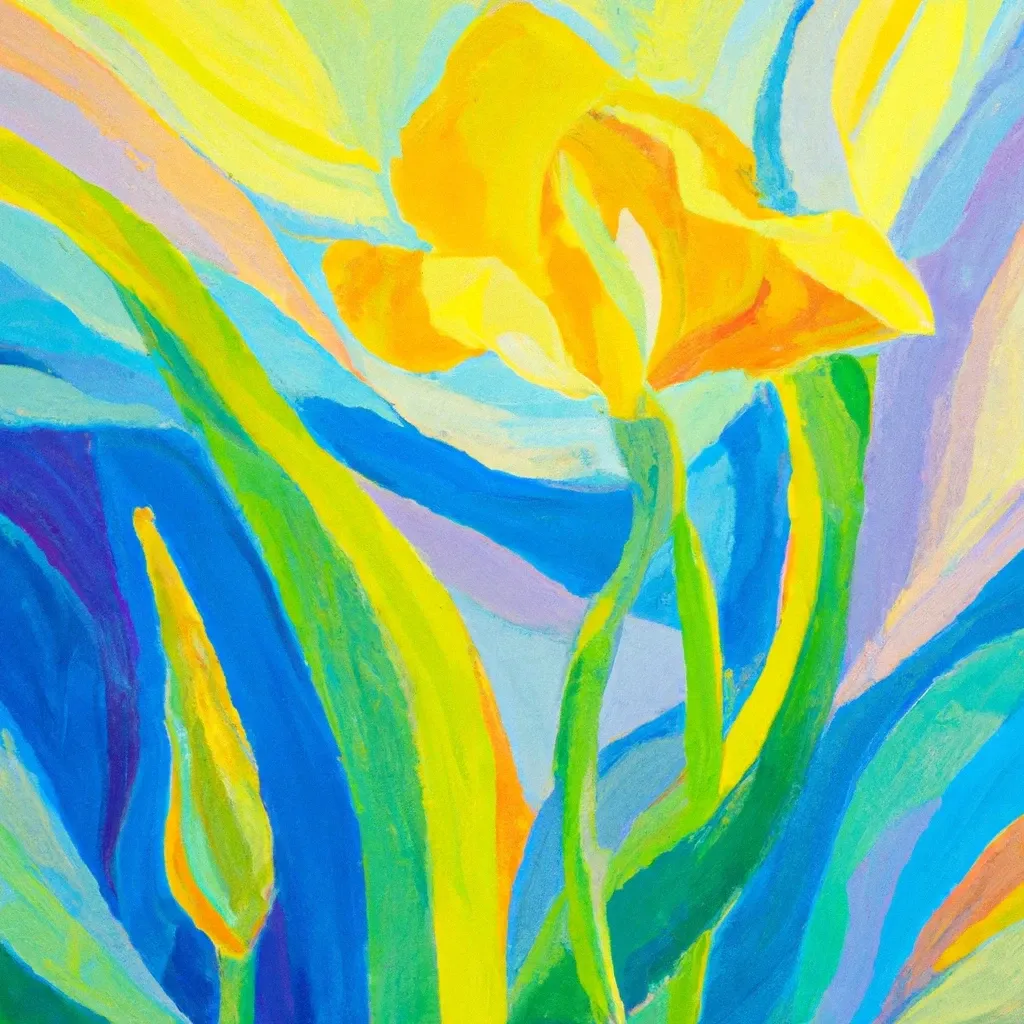
A New Paradigm: The AI Development Cycle.
The AI development cycle, marks a significant departure from traditional software development. It's data-driven, involves iterative model training, produces probabilistic outputs, and often continues learning post-deployment. This paradigm requires UX designers to be involved end-to-end, collaborate closely with data scientists and engineers, and create adaptive interfaces that can evolve with AI capabilities.
If we think about the start of product development, we begin with a business problem to solve. It's the same process for ML. We are leaning on data much earlier in the process, as it shapes throughout the project.
Key Considerations:
- Data-Driven Development: Unlike traditional software where logic is explicitly coded, AI systems learn from data. This shift requires UX designers to be involved in initial data selection and curation, to post-deployment monitoring and refinement, as these choices directly impact user experience. Designers need to understand technical constraints and opportunities to create effective AI-powered interfaces, and address bias and ethical concerns early in the design process.
- Iterative Model Training with probabilistic outputs: AI models undergo continuous refinement through training. Providing probabilistic results rather than deterministic ones. UX designs must be flexible enough to accommodate evolving AI capabilities without requiring complete redesigns. As AI systems become more complex, designers play a crucial role in making AI decisions understandable and trustworthy to users.
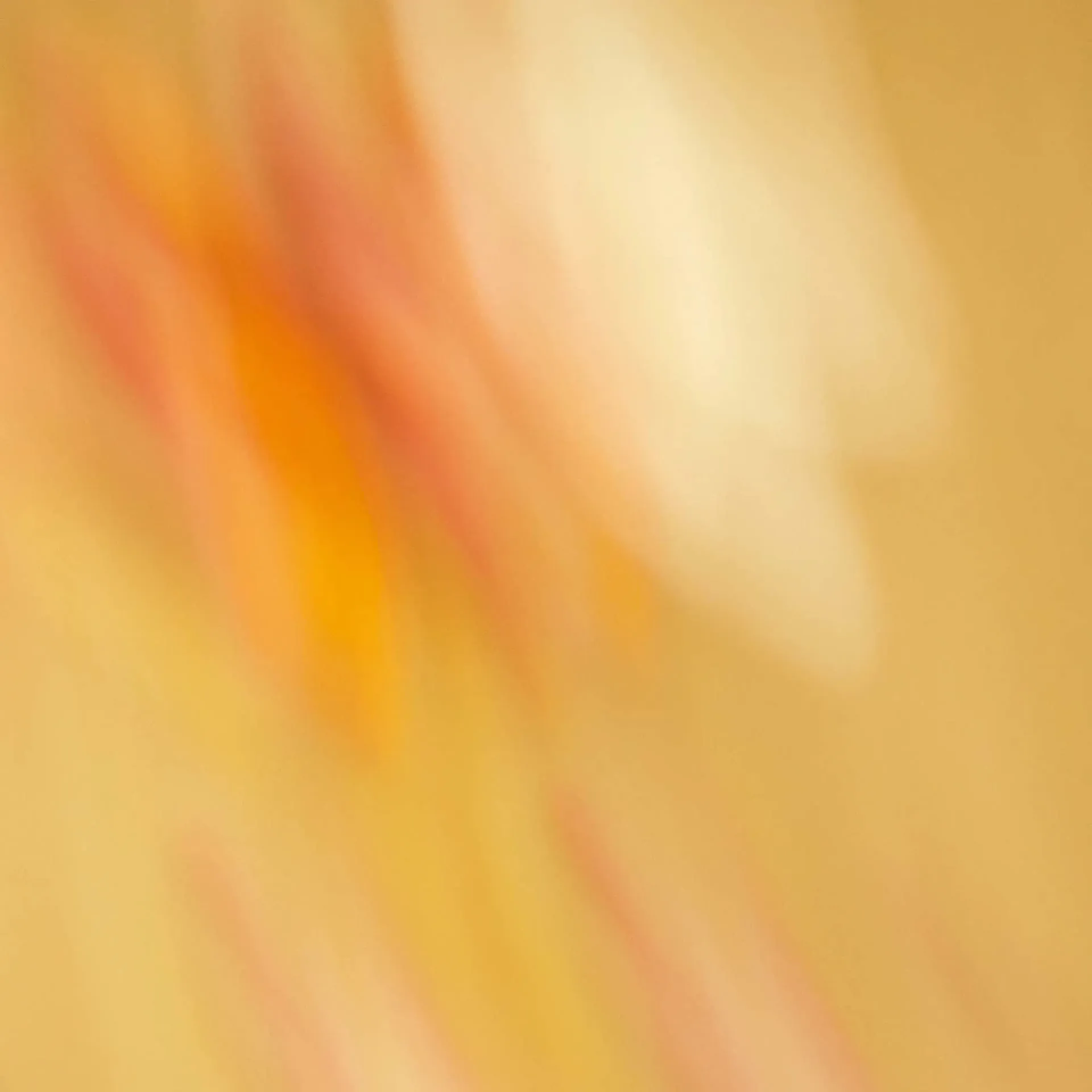
Value Migration in AI: Generalist vs. Specialist Model
Value in AI is migrating from general-purpose models to specialized, domain-specific applications. The focus is shifting from technical capabilities to user value, and from data quantity to quality. As UX designers, we must develop vertical expertise, design for contextual intelligence, and critically think in terms of ecosystems and value-driven metrics.
Understanding the trade-offs between generalist and specialist AI is crucial. UX design should align with use cases, manage user expectations, consider hybrid approaches, ensure scalability, maintain contextual relevance, handle errors robustly, and leverage both approaches for effective personalization.
- From General to Specialized: AWS's Bedrock, and Chatgpt are general purpose LLMs. While there is value in a broad foundational model, domain-specific LLM and applications provide the fine-grain, tailored experiences that are effective for users. We need to develop deep domain expertise to create AI-powered experiences that truly resonate with users in specific industries or use cases.
- From Data Quantity to Quality: We are shifting from amassing large quantities of data to curating high-quality, relevant datasets. Designers need to advocate for data that will lead to meaningful experiences.
- Success metrics for AI-powered features should be tied to user value and business outcomes, not only technical performance. Our designs should focus on how AI can augment and enhance user capabilities, rather than simply showcasing AI technology.
Key Considerations:
- Clearly define the scope of AI functionality in your product, avoiding the temptation to over-promise with generalist AI or over-restrict with specialist AI. Determine whether your product needs the versatility, precision, or hybrid approach based on user needs and use cases.
- Design interfaces that communicate the AI's capabilities and limitations
- Scalability and flexibility with design systems with AI components that evolve between specialist and generalist capabilities and user needs to develop over time.
- Contextual Relevance. Ensure that AI outputs, whether from generalist or specialist systems, are presented in a context-appropriate manner within the user interface.
- Error Handling. Design robust error handling and fallback mechanisms, recognizing that generalist and specialist AIs may fail in different ways. Design interfaces that can accommodate both narrow, specific AI outputs and broader, more open-ended interactions. Is AI operating as a generalist or specialist in different contexts, helping users form appropriate mental models.
- Personalization. Leverage the strengths of both approaches to create personalized experiences that feel both broad and deeply relevant to individual users. Provide appropriate levels of user control, allowing users to switch between more generalist or specialist modes of interaction when relevant.
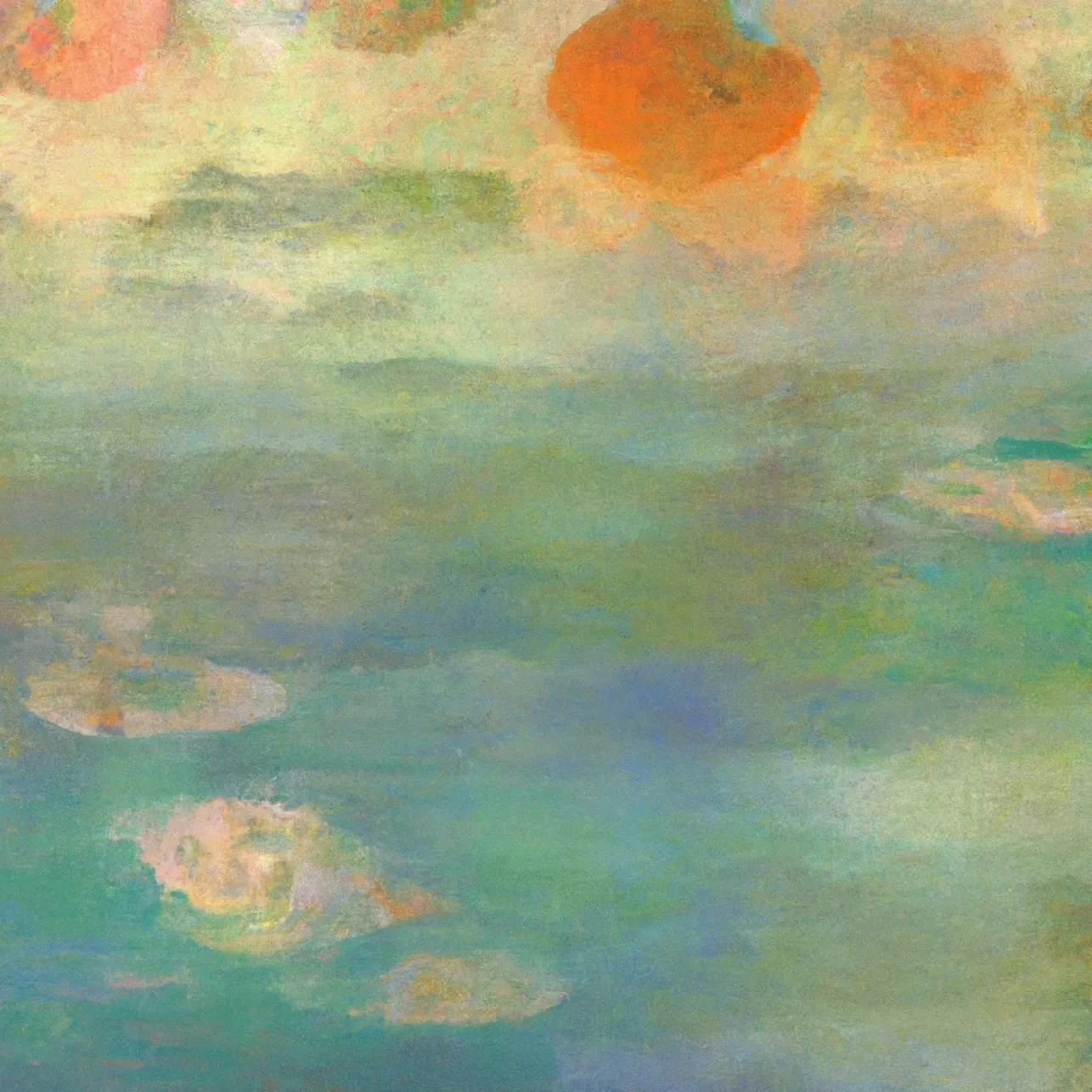
The Role of Design in AI Development
Designers now play crucial roles in data processing and feature selection, model development and experimentation, and system development and debugging. Our role is to bridge the gap between complex AI capabilities and intuitive, valuable user interactions. This expanded involvement challenges us to deepen our technical understanding while staying true to core design principles of usability, accessibility, and user-centeredness.
Key Considerations:
- Data Processing and Feature Selection:
- Designers help identify which data attributes are most relevant to user needs.
- We advocate for diverse, representative datasets to mitigate bias. Our input ensures that selected features align with user mental models and expectations.
- Model Development and Experimentation:
- We define objectives and reward functions in reinforcement learning.
- Our insights help align model outputs with intended user outcomes. We provide context for how model decisions will impact the overall user experience.
- Design, System Development and Errors:
- Designers help balance precision vs. recall trade-offs based on user needs and potential impacts.
- We design interfaces that accommodate the probabilistic nature of AI outputs for errors that are accessible and understandable to users. We design fallback experiences, progressive disclosure mechanisms, to handle complexities.
Our expanded responsibilities:
- Deepening Technical Understanding:
- Develop a solid grasp of AI/ML fundamentals, including data processing, model training, and evaluation metrics. Understand the capabilities and limitations of different AI technologies to inform design decisions.
- Stay updated on emerging AI trends and their potential impact on UX.
- Active Participation in AI Development:
- Engage in data selection and curation processes to ensure relevance to user needs.
- Contribute to defining model objectives and evaluation criteria.
- Collaborate in feature engineering to align with user mental models.
- Participate in model testing and refinement from a UX perspective.
- Bridge the gap between design, data science, and engineering teams.
- Facilitate communication between technical and non-technical stakeholders.
- Collaborate closely with product managers to align AI capabilities with business goals and user needs.
- Balancing Technical Capabilities with User Needs:
- Act as the user advocate throughout the AI development process.
- Translate complex AI capabilities into intuitive, valuable user experiences.
- Design interfaces that make AI functionalities accessible without overwhelming users.
- Strategic thinking & adaptive design approach:
- Contribute to product strategy by identifying opportunities for meaningful AI integration.
- Help shape AI roadmaps that balance technical feasibility with user value.
- Advocate for responsible AI development aligned with company values and user expectations.
- Develop flexible design systems that can evolve with improving AI capabilities.
- Create prototypes that can simulate AI behavior for testing and iteration.
- Design for graceful degradation when AI systems fail or produce unexpected results.
- Ethical AI Implementation:
- Proactively identify and address potential biases in AI systems.
- Design for transparency and explainability in AI-driven features.
- Ensure AI implementations respect user privacy and agency.
By embracing these evolving responsibilities, we as UX designers can play a pivotal role in shaping the future of AI-powered products. Our unique perspective ensures that as AI capabilities advance, they remain grounded in human needs and values. This evolution challenges us to grow beyond our traditional skill sets, but it also offers an exciting opportunity to have a profound impact on how AI technology is integrated into people's lives.
Have a great week ahead,
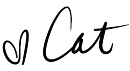